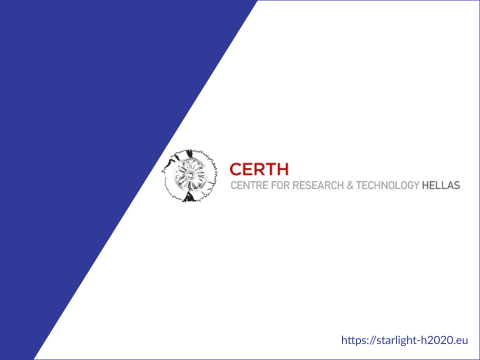
An update on Work Package 6, led by the Centre for Research & Technology, Hellas (CERTH).
Author: Michalis Lazaridis, Research Associate, Visual Computing Lab (VCL) @CERTH/ITI
One of the strategic goals of the STARLIGHT project is to reinforce and extend the capabilities of LEAs to effectively exploit AI for predicting, anticipating, preventing, detecting, and investigating criminality, terrorism, and monitoring borders, as well as for protecting digital infrastructures from cyber threats.
Especially in work package 6 of STARLIGHT, a wide list of AI tools in different readiness levels has been introduced so far and is expected to be delivered during the project’s lifetime. These tools are either being designed and developed within STARLIGHT or adopted, adapted, and improved from relevant past and current projects, such as ASGARD, DANTE, TENSOR, CONNEXIONS, AIDA, GRACE, and many others.
The tools cover several topics:
Discovering online content involves investigating AI-based strategies for discovering content relevant for a specific topic or purpose, as well as developing a crawler that can use such strategies to achieve focused crawling from the surface or deep web. The purpose is to allow for faster, more efficient retrieval of more relevant documents when information on a certain topic needs to be gathered.
Gathering sensor data from robotics and IoT systems involves the intelligent management of sensors and data-gathering devices. The data has to be assessed for variety, quality, and relevance during the sensing phase in order to facilitate the analysis in later phases.
Filtering and pre-processing data covers fast triage, data deduplication, and advanced data enhancement capabilities for determining which data needs to be analysed in detail and with what priority. This will lead to a reduction in the amount of data to be processed by investigators or subsequent processing methods and an enhancement of the multimedia data.
Detecting and identifying persons is related to human (re)identification exploiting visual information. Towards this objective, activities like face detection, tracking, person (re)identification, and verification are performed. Re-identification can be enhanced by analysing soft biometric features such as clothes, height, weight, age, gender, etc.
Detecting abnormal events covers detecting suspicious behaviours of individuals or crowds in several source types. Subtasks include the detection, tracking, and counting of persons, vehicles, or objects of interest; acoustic scene interpretation; the analysis of infrared or thermal camera content; and the automatic analysis of crowd behaviour.
Identifying objects of interest is related to analysing multimedia content to automatically extract and identify concepts of interest while filtering out irrelevant material and identifying potential intelligence.
Analysing text is related to converting unstructured multilingual textual data into knowledge that can be interpreted and further analysed. Subtasks include machine translation, named entity extraction, and concept identification.
Identifying anonymous speakers and writers covers audio and textual-based identity matching, which is devoted to revealing anonymous speakers or writers identities through the matching of voice characteristics, speaking styles, and writing styles.
As a further ongoing step, a match is created between the tools introduced in this work package and the LEA-defined scenarios and use cases, broken down into basic functionalities. This procedure is performed not only as a static mapping exercise between tools and functionalities but also as a dynamic matching exercise during the project’s tool fests between technical partners and LEAs. Following the matching step, the tools are iteratively redesigned, developed, and evaluated during the project’s co-development cycles.
This live procedure of use case redefinition, tool matching, and adaptation is the actual roadmap to achieve the final target, which is to provide opportunities to LEAs to exploit trustworthy, transparent, and human-centric AI tools and solutions in their operational work.
The Visual Computing Lab (VCL) of the Information Technologies Institute (ITI) is part of the Centre for Research and Technology Hellas (CERTH). The focus of the Visual Computing Lab is to develop new algorithms and architectures for applications in the areas of 3D processing, image and video processing, computer vision, pattern recognition, bioinformatics, and medical imaging.